Case Study
Predictive Maintainence
Machine breakdown affects the productivity of operations especially when the downtime is significant. This can be costly and affects the profitability of a business. We worked with a leading industrial manufacturing company to build an MVP that used AI to predict when an asset / machine was likely to fail so that necessary measures can be taken.
- Successfully able to predict failures before they happen with an accuracy of 91.4%
- The prediction capability results in direct reduction in maintenance costs
- Reduced downtime and machine failure rates
Challenge
A leading industrial manufacturing company had been using preventive maintenance for their electric motors. Preventive maintenance refers to a type of maintenance that you regularly perform on a machine to reduce the chance of it breaking down. Preventive maintenance aims at producing outcome-focused results for operating and maintaining based on the analysis. There are two ways for performing preventive maintenance, time-based and usage-based preventive maintenance.
On the other hand, reactive maintenance also known as Run to Failure is a simple method to execute. Under this strategy, you allow the machine to operate till it breaks down and then you perform the maintenance. However, you need to have a plan beforehand for the maintenance to reduce production risks and reduce unnecessary maintenance costs.
This strategy came at a huge cost of capital and human resources and the company wanted to experiment with predictive maintenance and see if an MVP can help them better predict when the electric motors were likely to fail, reduce downtime and reduce the overall cost of maintenance. There are primarily two potential failure modes that affect electric motors, electric winding failure or bearing failure. Each type of failure mode can be detected and predicted, enabling maintenance to plan and schedule removal of the motor to avoid unwanted downtime. Early detection of the problem is the easiest way to avoid such issues. One of the ways to do that is through predictive maintenance. Predictive maintenance refers to a method of using machine learning to decide the optimal time of service for the machine. This assists in the maintenance of the machine and reduces the machine downtime by servicing the machine just before it breaks down.
We started collaborating with the client and the key requirement of the project were
- Develop a predictive maintenance system that could outperform existing preventive maintenance strategies
- Process large volumes of data in real-time
- Create real-time alerts for maintenance personnel
The Solution
Predictive maintenance relies on three main elements for its success.
Availability of data
The availability of data involves collecting historical data on the performance of your machine. Historical data consists of collecting data over the degradation process of the machine. We collected data from various different data historians and production systems. Once this data was collected, we went through a pre-processing stage with the following steps
- Data cleaning : Cleaning the data involved removing wrong values (negative frequency values etc) and irrelevant data
- Data Imputing : This is the process of replacing missing fields in time series data and we used a number of different techniques to do this
- Data structuring and normalisation : The data was structure in a time series format so that it could show a continuous flow
Framing of the problem
Once the data was prepared we performed an extensive analysis using various data science techniques to understand correlation between different features and the failures. There are several questions that you have to ask when framing the issue. These questions include
- What type of output do we want the model to produce?
- What kind of feature engineering will be involved?
- Does the data have all the records of the events?
- What is the time frame for a model to indicate a failure?
- What are the performance targets for the optimisation of models?
The answers to these questions are crucial in deciding which model and optimisation strategy will suit the objectives and requirements of the project. Under predictive maintenance, different modelling strategies exist and included a range of different models in our PoC including LSTMs (Long Short-term Memory), ARIMA etc which we will discuss in the next section.
After the design phase was completed, we start training various models on the dataset and our final machine learning system comprised of an ensemble with various models and strategies. Once our prediction models were trained for different strategies, we evaluated these on test dataset. Prediction evaluation involves analysing the outcome of the problem framing and coming up with the appropriate measures. Such measures include analysing the cost for maintenance and potential savings that can be generated from early prediction and diagnosis of assets.
The complete MVP was deployed in the cloud with real-time alert functionality and integrated with the client’s historian. The complete architecture can be seen in the diagram below.
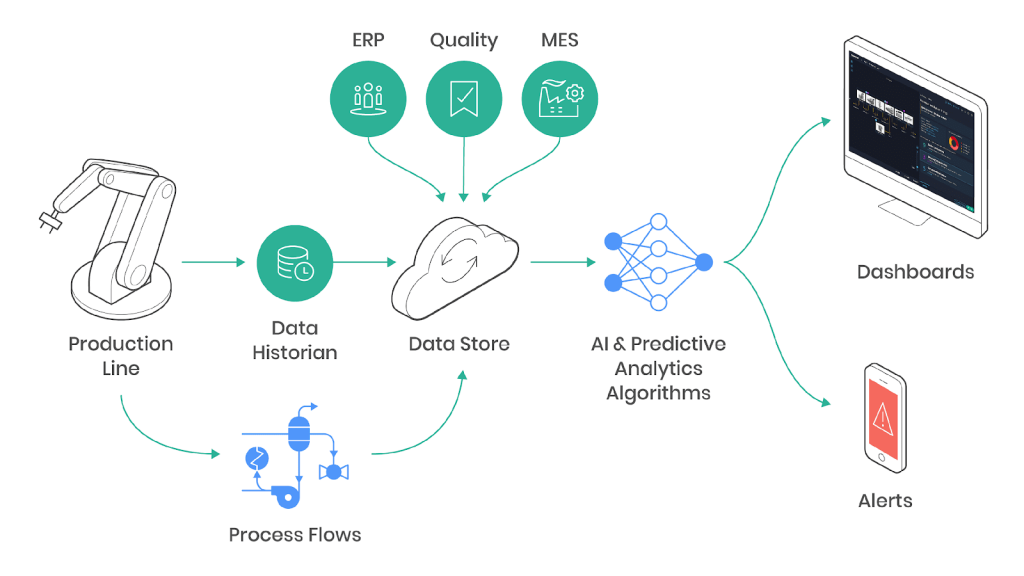
Technical Bit
We trained a range of different models which serve different purposes. Below are some of the strategies used for training and testing of different kind of models that together make up our machine learning system for IoT Predictive Maintenance.
- Flagging of unusual behaviour : Under this strategy we train anomaly detection models which detect abnormalities in assets and sensor networks
- Regression model strategy for predicting the Remaining Useful Lifetime (RUL). Here, we need to ensure that you have all the data, label each event and have several events of each type of failure. These models at any point in time predict the remaining useful life of an asset.
- Survival model strategy to predict the failure probability over a given period. This strategy is best if you want to find the degradation process of the machine and its failure probability. This strategy requires static data and failure time information of each machine
- Classification model strategy that is useful in predicting the failure of a tool during a given time frame. Here, the description of failure is on a time frame and it deals with multiple types of failures.
Results
Training and deploying predictive maintenance solution yielded phenomenal results for the business in terms of cost savings and increasing production output.
The use of traditional maintenance implies that you have to wait for a problem to occur before fixing it. This means that during the maintenance period, the machine won’t be operating which leads to loss of revenue during that period. On the other hand, predictive maintenance identifies when the machine will have to undergo maintenance. This gives operators time to make alternate arrangements for maintenance.
With preventive maintenance solutions, businesses have to schedule maintenance even when it is not needed. This results in unnecessary costs and wastage of resources. On historical datasets, our system predicted a failures before they happened with a 91.4% accuracy. With this accuracy from our ensemble system, we were successfully able to deploy the MVP and are currently in the process of measuring the performance on realtime data with larger volume and scale. Once the initial positive results have been assessed then a wider rollout with more assets and machines will occur.
Interested in finding out how Intelligent Predictive Maintenance solutions can help your business save money?